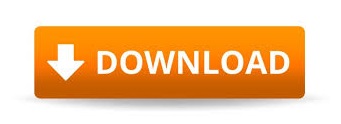
Therefore, an important question is to evaluate what is the added value of including genomic information in multi-trait genomic prediction. Multi-trait prediction allows to use information simultaneously from relatives and from different traits. These might be closely linked traits, for example somatic cell count as indicator trait of mastitis, or the same trait recorded in a different environment or country. One effective strategy that has been used to deal with such traits in the past, without using genotypic information, has been the implementation of multi-trait prediction with indicator traits that are easier or cheaper to record. īy putting emphasis on loci that are closely linked to causative loci, genomic prediction holds the promise to be particularly beneficial for selection on traits that are difficult or expensive to measure, that are sex-linked, or that are expressed late in life. An added benefit of such integrated marker selection procedures is that posterior distributions are provided for the probability that a locus affects a trait, and these can be used for QTL (Quantitative Trait Loci) mapping purposes. whether a locus affects the trait or not, is often integrated in the sampling process using model selection. Reduction of dimensionality of the markers, i.e. Most of the proposed models try to reduce the effective dimensionality of the marker data, since the number of markers is typically much larger than the number of phenotyped animals in the reference population. Many different models have been proposed to simultaneously estimate marker effects. The key principle for all these applications is the simultaneous estimation of all genome-wide marker effects based on a reference population with known phenotypes. Genome-wide prediction is also being recognized as an important tool to predict phenotypes and genetic risk for diseases in other fields than animal or plant breeding. Genomic selection uses genome-wide dense marker maps to accurately predict the genetic ability of an animal, without the need of recording phenotypic performance of its own or from close relatives, such as sibs or offspring e.g. , and is an emerging alternative for marker-assisted selection in plant breeding. In particular, the application of genomic selection is becoming the new standard in animal breeding e.g. For different combinations of traits, the level of genetic correlation below which genotyping selection candidates is more effective than obtaining phenotypes for an indicator trait, needs to be derived considering at least the heritabilities and the numbers of animals recorded for the traits involved.ĭue to the availability of affordable genome-wide dense marker maps, the use of marker information in practical animal and plant breeding programs is increasing. However, when genetic correlations were higher than 0.5, using an indicator trait led to higher accuracies for selection candidates. When the indicator trait had a genetic correlation lower than 0.5 with the trait of interest in our simulated data, the accuracy was higher if genotypes rather than phenotypes were obtained for the indicator trait. For animals with phenotypes on an indicator trait only, accuracy increased up to 0.03 and 0.14, for genetic correlations with the evaluated trait of 0.25 and 0.75, respectively. Using multi-trait SNP-based models, the accuracy for juvenile animals without any phenotypes increased up to 0.10. In terms of accuracies for animals without phenotypes, the models generally ranked as follows: BSSVS > BCπ0 > G > A. For reasons of comparison, model A including pedigree but not SNP information was fitted as well. Model BSSVS sampled SNP effects from a distribution with large (or small) effects to model SNP that are (or not) associated with a quantitative trait locus. Model G and BCπ0 assumed that contributed (co)variances of all SNP are equal. Three (SNP) Single Nucleotide Polymorphism based models were used. The objective of this paper was to develop three models that would permit multi-trait genomic selection by combining scarcely recorded traits with genetically correlated indicator traits, and to compare their performance to single-trait models, using simulated datasets. It holds the promise to be particularly beneficial to select for traits that are difficult or expensive to measure, such as traits that are measured in one environment and selected for in another environment. Genomic selection has become a very important tool in animal genetics and is rapidly emerging in plant genetics.
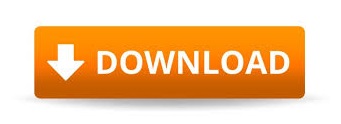